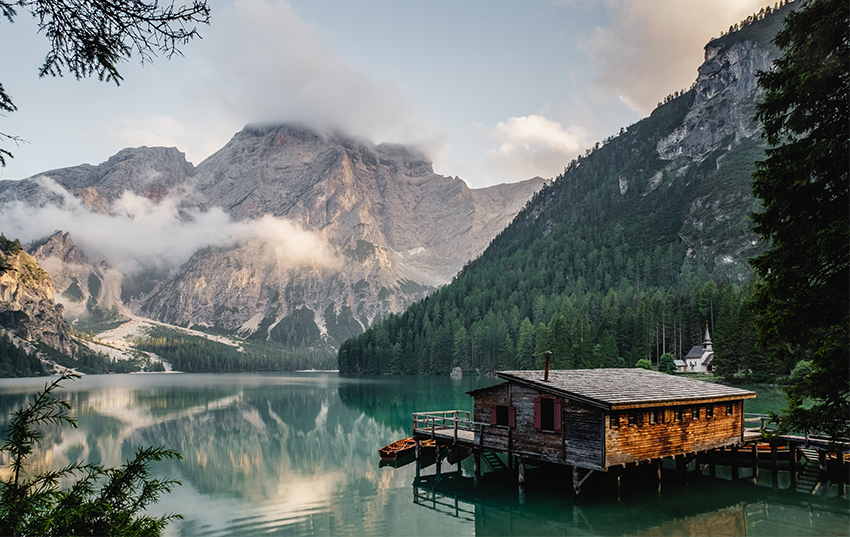
The concept of a ‘data lakehouse’ is set to gain more ground in 2021, even though the term itself divides opinion among data storage and management services providers, according to 451 Research analyst Matt Aslett.
A data lakehouse is a concept that combines a data lake’s low-cost cloud storage capability with a data warehouse’s structured data management and processing functionality.
The community of vendors offering services that fit the general description of a data lakehouse is growing steadily. But they are offering the service under different names, which has been the source of some confusion.
The many names of data lakehouse
Several vendors have started adding structured data processing concepts and functionalities to cloud storage itself, instead of exporting it to external data warehouses. The approach brings in a clear performance and efficiency advantage as businesses continue to collect and store large volumes of unfiltered data.
But what are they calling this phenomenon?
The term ‘data lake’ was first coined 10 years ago by the cofounder and then CTO of Pentaho, James Dixon.
Even though Amazon and Snowflake had already started using the term ‘lakehouse,’ the clearest endorsement came from Databricks in a January 30, 2020, blog.
The blog described a data lakehouse as “a new, open paradigm that combines the best elements of data lakes and data warehouses.”
Data lake vs. data warehouse
Today, organizations deal with large volumes of data, which can be structured, semi-structured and unstructured, and can come from various sources.
A data lake offers a single, open and cost-effective ecosystem to store large volumes of raw, unfiltered data. Such functionality is well-suited for continuously evolving business needs. However, results have been mixed.
The data lake has largely failed to live up the hype due to lack of appropriate functionalities for data processing, management and governance. Retrieving the unfiltered data stored in a data lake often becomes challenging for general business or self-service users. The issue is further compounded amid increased regulatory scrutiny of data storage around the world.
On the other hand, traditional data warehouses provide strong reporting and data analysis capabilities, but they lack the ability to handle semi-structured and unstructured data like text, images, video and audio. As a result, data warehouses are inflexible and costly.
A common workaround for these shortcomings is to connect a data lake to several external data warehouses and other specialized systems. However, simultaneously managing multiple systems is complex, inefficient and costly.
Who is swimming in the data lake?
As discussed earlier, there are several vendors that offer what can be loosely defined as a data lakehouse, even though they do not quite endorse the term. Here are a few:
Amazon: Redshift Spectrum enables clients to query structured and semi-structured data across their data warehouse, data lake and operational databases, without having to load the data into Amazon Redshift tables. It allows multiple clusters to query the same dataset from S3 cloud, a data lake, without the need to make copies. It first used the term ‘lake house’ for Redshift Spectrum in 2019.
Databricks: Launched in April 2019, Delta Lake provides a structured transactional layer with support for ACID (atomicity, consistency, isolation, durability) transactions, updates and deletes, and schema enforcement. In July 2020, it added to Delta Lake a high-performance query engine, Delta Engine, and later added SQL Analytics, which is compatible with business intelligence visualization offerings such as Microsoft’s PowerBI and Salesforce’s Tableau.
Google: BigQuery is Google’s cloud-based enterprise data warehouse that offers functionalities like transaction support, schema enforcement and governance and storage, decoupled from compute. While Google does not identify it as a data lakehouse, it certainly qualifies as one.
Microsoft: Azure Synapse Analytics combines Azure SQL Data Warehouse functionality with big-data processing, data integration tooling and the ability to leverage Azure Data Lake Storage as a common storage layer.
IBM: Cloud Data Lake Reference Architecture combines its SQL Query, Db2 Warehouse and analytics from Watson Studio and Watson Machine Learning with IBM Object Storage.
Cloudera: The company offers structured data warehousing, machine learning and other services for management and analysis of data in object storage.
Snowflake: While Snowflake in 2017 became arguably the first vendor to use the term lakehouse, the company itself prefers calling it a data cloud. The data-warehousing provider recently started promoting its data lake capabilities. It has added early preview support for the processing and analysis of unstructured data to its existing native support for semi-structured data, as well as the use of external tables to enable the analysis of semi-structured and unstructured data in external resources.
Want insights on data platforms and analytics trends delivered to your inbox? Join the 451 Alliance.