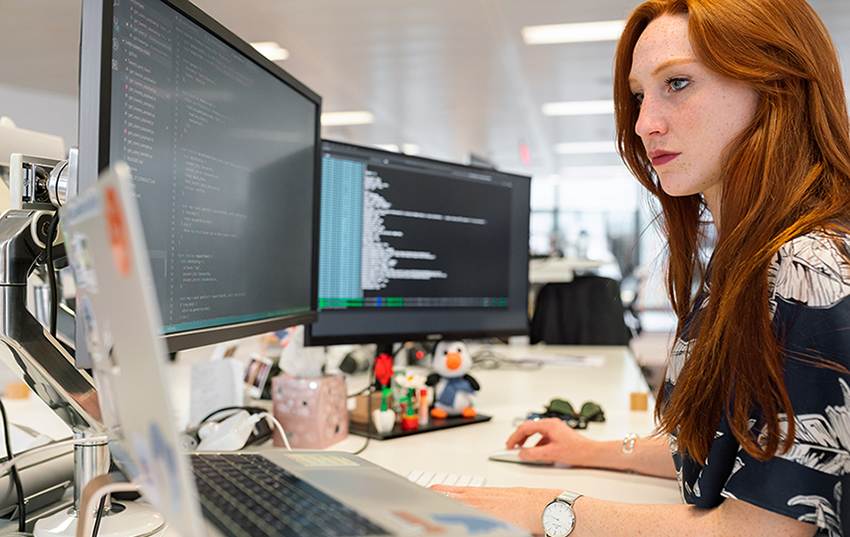
One day, AI infrastructure and IT infrastructure will be one and the same. Right now, they are not, and that is why we pay particular attention to how organizations use infrastructure to help their AI projects scale. For those implementing AI in the form of machine learning, deep learning and its other variants, IT infrastructure is top of mind and requires special attention in terms of what technology is acquired and how it is paid for.
A 451 Research survey explores these ideas, and includes direct input from 700 AI decision-makers across the U.S. and U.K. The insight provided reveals many trends and developments in the market, including the ever-increasing volumes of data and models requiring management, a shift toward both the cloud and the edge, and several hurdles that need to be overcome to get AI projects from concept into production.
The Take
The adoption of AI is driving changes in the infrastructure that underpins it, both in terms of technical changes from chips up to the cloud, and in the way organizations plan and purchase such infrastructure.
It requires a focus on the technologies needed to accelerate AI and enable it to scale — all of which costs money. AI has unique infrastructure requirements, and organizations engaged in AI spend significant amounts of their IT infrastructure budgets on it — with many doing so on a scale-on-demand basis. What they are looking for varies, but the ability to move large volumes of data around is key, and means that higher-performing networks are again atop organizations’ wish lists, as they have been in past years of this survey.
Sustainability also matters, and most organizations are willing to invest in sustainable AI/machine learning (ML) infrastructure. Although cloud has featured in the AI adoption cycle, AI inference at the edge — in all its forms — is what people want to do in the future, so it will likely feature as a prominent factor in the planning of future AI infrastructure.
Summary of findings
AI infrastructure planning requires a different approach to the one used for IT infrastructure. Fifty-nine percent of organizations plan AI differently than other IT infrastructure. While there are many reasons for doing so, the most common are network requirements, storage performance and different technical/hardware requirements. Virtually all organizations agree (99%) that it is important for AI-driven applications to be able to integrate with existing infrastructure. However, difficulty integrating AI with existing infrastructure is the top selected primary reason for planning AI differently, and is a particularly motivating factor in the healthcare, telecommunications and manufacturing sectors.
AI/ML workload demands on infrastructure are ever-increasing. Eighty-one percent of respondents expect their AI/ML workload demands to increase over the next two years, compared with 76% of respondents to the same survey in 2021. This is especially the case for larger organizations — 89% of organizations with more than 10,000 employees expect AI infrastructure demands to increase.
Many IT environments are not prepared for the future. Sixty-five percent of IT environments require some level of upgrade to meet future infrastructure demands. For 40%, these changes are minor; however, one-quarter of IT environments require moderate to major upgrades.
More models are being deployed into production. The average number of models in production has risen significantly this year to 3,444, compared with just 1,991 in 2021. Meanwhile, the average number of models in proof of concept has decreased. This change is particularly apparent when looking exclusively at organizations that have ML in production, reflecting the steady maturation of AI/ML initiatives as a whole.
Data volumes rise with maturity. The amount of data used for building models and making predictions is greatest among those that have been doing AI the longest. Those that began developing their first ML project more than five years ago on average use 107 PB of data for building and training models, and 155 PB for inference. A considerably higher proportion of those that began ML development less than six months ago expect a significant increase (50% or more) in the amount of data used for building and training models (32%) than those that began development more than five years ago (19%).
Data, data everywhere, but not a byte to train. Slightly more than half (51%) of organizations have just the right amount of data to build and train their AI/ML models, and two in five have more than enough. However, having data doesn’t make it accessible — 65% of organizations have difficulty getting access to the data they need for their AI/ML workloads, and this difficulty is only compounded for those with larger data volumes.
“Scale on demand” remains the most popular approach to AI infrastructure spending. A plurality (47%) of organizations use “scale on demand” as their purchasing strategy for AI/ML infrastructure, compared with 39% that plan in advance and 11% that use a designated amount.
On average, organizations spend 43% of their IT infrastructure budget to support AI. This jumps to 51% for those with ML in the production stages and 60% for companies younger than 10 years old.
Spending on AI infrastructure has remained steady, but costs rise with maturity. On average, over the past 12 months, organizations have spent about $1.4 million on AI/ML infrastructure. This is slightly less than the 12 months prior ($1.5 million), suggesting that the cost of AI has remained steady, if not come down marginally. However, spending creeps up as organizations reach later maturity stages. Those with ML in production spent an average of $1.6 million on AI infrastructure in 2021, compared with $1.3 million spent by those with ML in proof of concept. Those that began development in the past two years have spent $1.1 million, while those that began development more than five years ago spend about $1.9 million.
There are plenty of infrastructure resources that organizations need, and higher-performance networking is at the top of their wish lists. Forty-five percent of organizations identify higher-performance networking as a resource they need to improve their AI/ML workload performance, and 23% say it is essential. Other high-ticket items include more scalable, higher-performance storage (36%); accelerators in the cloud (33%); and faster standard servers (32%).
Low-code tools are a growing enterprise asset. Fifty-nine percent of organizations with AI/ML initiatives are currently using low-code tools, and an additional 29% plan to within the next year. Those currently using low-code tools cite ease of integration (37%), increased scalability (36%) and faster time to deployment (33%) as their primary benefits.
Cloud by necessity, but edge is what organizations want. Public cloud remains the most popular primary AI workload venue for storage, training and inference (all at 45%); however, 95% of organizations claim that they would prefer to do more training and inference on the edge, but are limited by factors including storage capacity, budget and compute performance.
Sustainability matters. Overall, 85% of organizations claim they are willing to pay for sustainable AI/ML infrastructure; 30% see it as essential and are willing to pay a premium for it. This is especially the case in the energy, oil and gas sector, where 44% of respondents see sustainability as essential.
Want insights on AI trends delivered to your inbox? Join the 451 Alliance.