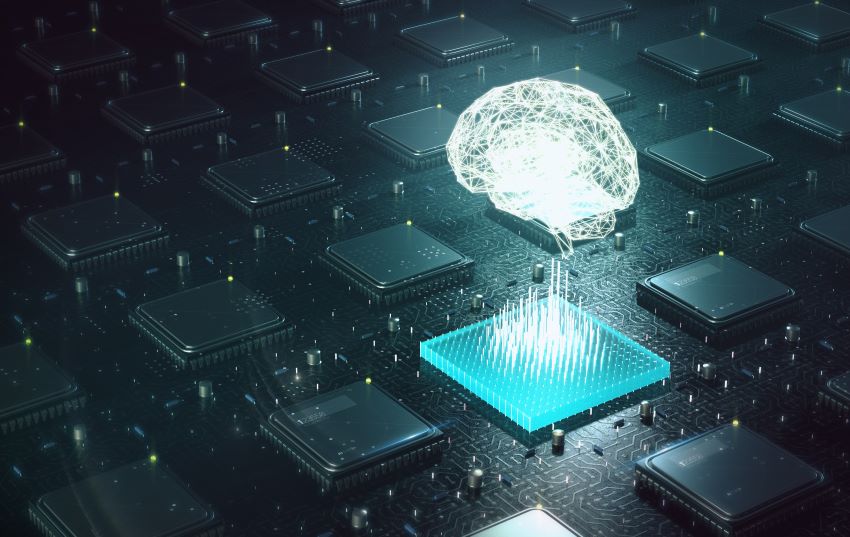
By now, almost everyone understands the massive impact artificial intelligence (AI) will have on business processes. Through automation and optimization, the technology will digitally transform a variety of legacy processes, from back-end business systems and customer service to supply chains and R&D.
What many business leaders do not yet grasp is the infrastructure demands the technology will place on their IT environments, be it public cloud or on-premise hardware. According to a 451 Alliance survey of AI adopters, only 27% of enterprises have both developed and implemented a strategy around their IT infrastructure for AI. Organizations will need to do better in order to ensure their AI implementations are successful in the long term.
Only 27% of enterprises have both developed and implemented a strategy around their IT infrastructure for AI.
One important consideration for any enterprise looking to adopt AI is the location of AI workloads within the enterprise network. In the paradigmatic AI scenario, a machine learning expert will aggregate data from disparate sources into a central repository, usually a cloud environment, in order to train a model. That model is then deployed either centrally or in an edge environment, depending on the needs of the AI application. This framework, however, is not universally applicable.
We asked AI adopters about where workloads for each step of the AI process – data preparation, training and inference – occurred within the enterprise network, and the results are displayed below.
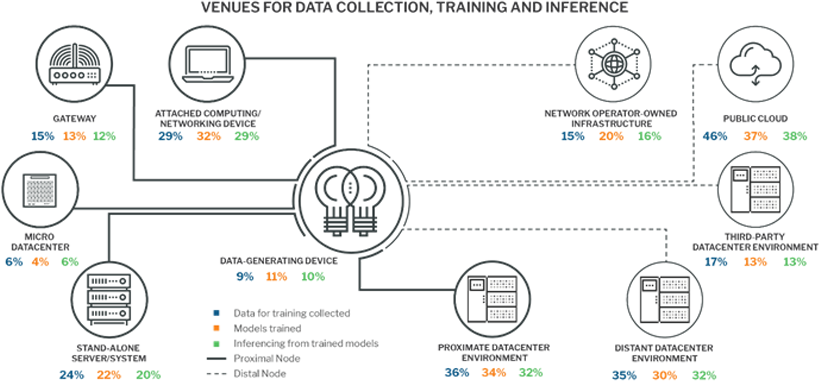
The diagram demonstrates that public cloud is currently the most popular venue for all stages of the AI process. Almost half of AI adopters are using the public cloud for data preparation, and over a third leverage it for model training and inference.
Private data center environments – whether proximate or distant – are also popular compute environments for AI workloads, far outstripping the usage of third-party data centers. Overall, traditional data center environments are handling the bulk of AI workloads, which makes sense given the relative flexibility and compute-power of this infrastructure.
The edge, it seems, is still an emerging venue for AI workloads. Only a small percentage of enterprises have located workloads on data-generating devices or micro data centers. Instead, AI adopters are using stand-alone servers or attached compute in order to drive AI at the edge. These numbers may very well change as more edge-specific hardware comes to market to target AI use cases in which real-time decision making is paramount.
Considering where AI workloads will occur is a critical step in a company’s journey to AI adoption. Understanding the infrastructure needs will make the implementation process smoother, ensuring better time-to-value and higher success for this transformative technology.
Want insights on AI trends delivered to your inbox? Join the 451 Alliance.