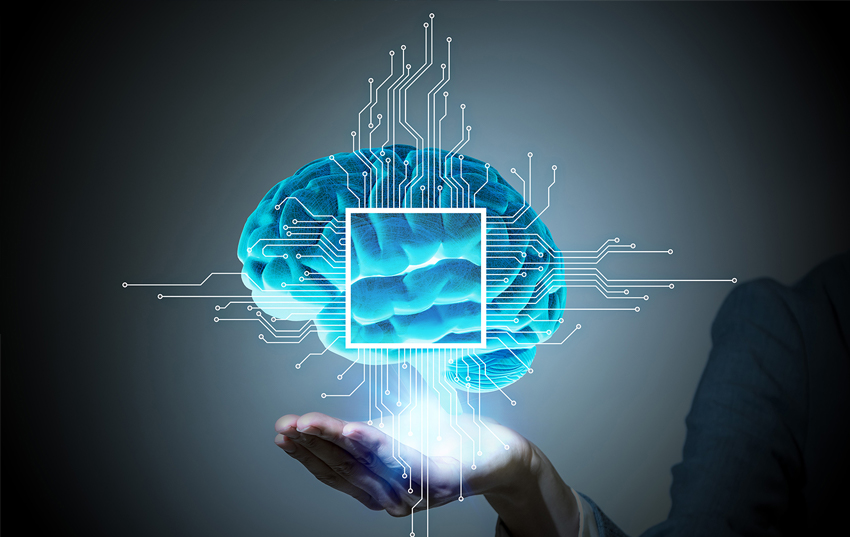
First off, thank you to everyone who attended the 451 Alliance webinar, The State of Enterprise AI in 2021, on Tuesday, February 16, 2021, where Research Director Nick Patience discussed new AI and machine-learning use cases across six major industries, and addressed current concerns about the infrastructure underpinning AI adoption at scale. Many great questions came in during the Q&A session, some of which we couldn’t address live. Here are some of the hard-hitting questions about AI, answered by the experts.
Where do you see AI having the biggest impact?
In a recent 451 Alliance survey, we asked respondents how much of an impact – if any – AI would have on their industry over the next two years. 54% of those in the financial services sector said AI would have a significant impact on their industry, and 77% anticipate its impact to be positive.
The financial services sector has much more readily adopted AI than some others, so these numbers come as no surprise. However, we can also look at impact in terms of growth potential. The healthcare and manufacturing sectors have arguably been sluggish in AI adoption, leaving the door open for greater impact down the line as adoption becomes more widespread.
There are several strong use cases in these sectors, such as patient data analysis and clinician workflow automation in the healthcare space, and quality assurance and predictive maintenance in the manufacturing space. Between the two, the manufacturing sector has made great bounds in shedding its laggard reputation, and we anticipate more growth here in the years to come.
How do AI concerns diverge by industry?
When it comes to adopting and deploying AI, budget limitations and skills shortages are generally considered the biggest challenges. But across industries, other challenges may be of equal or even greater concern. For example, according to 451 Alliance survey data, model explainability is the top concern for respondents in the software and IT sector, with budget limitations being far less paramount. Budget is a much bigger concern in heavily regulated sectors such as healthcare and government.
As for other industries, accessibility of data is a particular concern for those in retail and financial services, while building or obtaining appropriate algorithms is a major concern in the telco space.
How do you verify the competence of AI in new products?
This can be a tricky question to answer, as needs and success metrics vary widely from one business to another, and there’s no standardized method for testing AI competency across the board. That said, at 451 Research, we have a five-step process we walk businesses through, when they are considering ML, to determine if adoption is the right solution for addressing their business problem.
Begin by understanding the business problem and rules thoroughly. Before undertaking an ML project, it’s important to have a firm grasp of the problem you’re trying to solve; only then can you identify whether ML is a suitable solution.
The next step is having a decent amount of data that isn’t too siloed. The precise amount of data needed depends on the business problem and types of algorithms being used, but overall, a successful ML initiative requires data that is both accessible and available.
Following this, businesses want to identify good and bad outcomes. During the training stage, is the software conceptualizing patterns and associations that don’t exist in the real world? This is a problem called ‘overfitting,’ where models could be creating patterns from irrelevant information (or ‘noise’). In these situations, models perform well with training data, but poorly when applied in real-world scenarios – which is ultimately a bad outcome.
Finally, businesses should understand the likely impact on user experience. Once a prediction is made, is it clear what the next best course of action is? What is the confidence level of this prediction, and can it be trusted? Can the outcome be explained to non-technical users? These are all things for businesses to consider when evaluating their AI products.
How far away is artificial general intelligence?
There are some companies pursuing Artificial General Intelligence (AGI) – the idea we can match the highly adaptive and generalized intelligence that humans have in software and put it to work. We actually polled the audience with this question during the webinar and many of you had an optimistic outlook on the timeframe for AGI, some even expecting it to be reached within the next 5 years, which is incredibly optimistic.
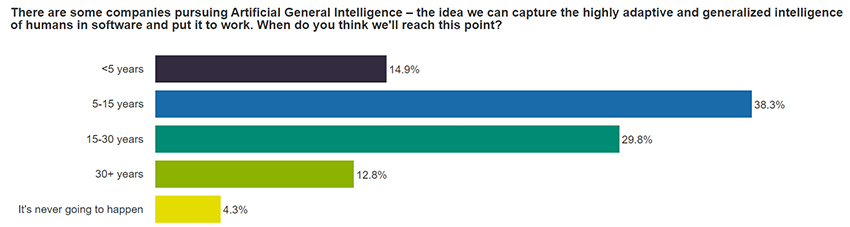
Though AI technology is advancing rapidly, we feel there’s still a ways to go in reaching AGI and therefore our estimated timeframe is somewhat longer, between 30 and 50 years.
Missed this webinar or want to watch it again? Members can access the recording here: The State of Enterprise AI in 2021.