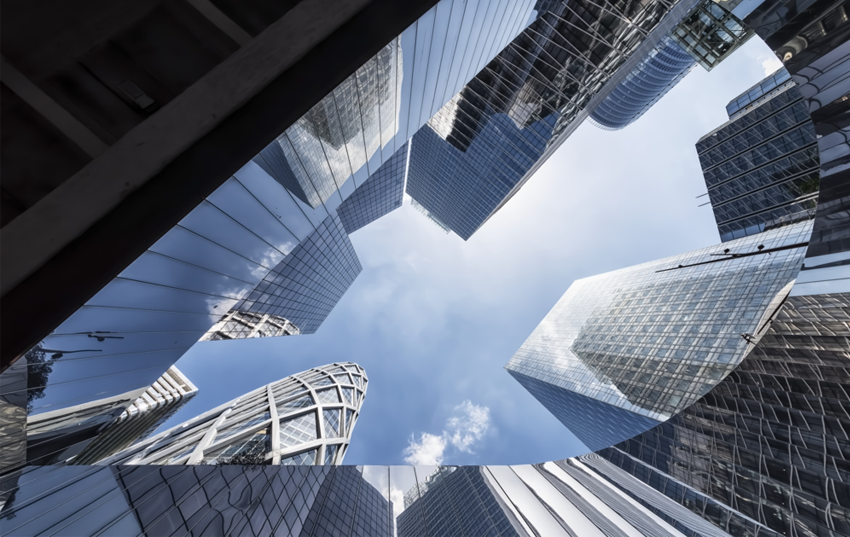
Generative artificial inteligence is the subject of immense hype in the enterprise IT industry, but our latest DevOps survey reveals some substance to the trend in software development, with nearly half of respondents (48%) reporting generative AI in use, discovery or proof of concept. Use is being driven primarily by higher-quality software, faster problem solving/issue resolution, more accurate data and improved developer productivity and experience. Key challenges of using generative AI in software development include data/IP sensitivity, measuring and proving effectiveness, and lack of vendor support, according to the survey.
The Take
Generative AI (GenAI) in software development holds the promise of further reducing toil and repetitive tasks for software developers with automation, so they are free to focus more on new features, products and innovation. However, like open-source software, DevOps and cloud native have shown, the promise comes with challenges as well.
Our DevOps, Generative AI in Software Development survey reveals a breadth of different GenAI technology in use beyond just ChatGPT (81%), including Bard AI (36%), JasperChat (18%) and others.
It also reveals most use of GenAI in software development centers on software testing (53%), security scans (43%), code review (38%) and code generation (36%).
We also found that GenAI actually serves as a driver of recruitment and retention (55%) of employees in software development, rather than decreasing it (14%).
Top among concerns around its use in software development are data privacy and security risks (45%), skills and training required (40%), and legal and regulatory compliance concerns (33%). Also concerning to survey respondents are loss of human interaction (33%) and loss of human creativity (31%).
Key Findings
Nearly half of organizations (48%) have GenAI in use or in discovery/proof of concept for their software development. While ChatGPT is the most popular GenAI technology for software development (81%), there is also significant use of Bard AI (36%) and several other technologies (Jasper Chat at 18%, AI2SQL and DialoGPT at 17%, Chatsonic at 15%, Socratic at 13% and LaMDA at 12%). This makes it clear that GenAI in software development is much broader than just ChatGPT.
GenAI is used and planned for most in software development for software testing (58%), security scans (46%), code generation (37%), document generation (37%), code review (37%) and code suggestions (33%). Expected benefits for those using GenAI in software development are higher quality software (65%), faster problem solving/issue resolution (59%), more accurate data (56%) and improved developer productivity and developer experience (both 54%).
At least in enterprise software development, GenAI is a driver of recruitment and retention of employees, with 20% of organizations reporting a significant increase and another 35% reporting a slight increase, while only 12% report a slight decrease and 2% report a significant decrease. Another 31% of organizations say GenAI has had no effect on their recruitment and retention of employees for software development.
The biggest challenges for those using GenAI in software development are data/IP sensitivity (41%), measuring effectiveness (39%), proving effectiveness (33%) and getting vendor support (31%). Top concerns are related and are identified as data privacy and security risks (45%), skills and training required (40%), and legal and regulatory compliance concerns (33%). Respondents also cite use of IT resources (33%) such as compute, CPU, storage and memory as a top concern.
Why enterprise organizations are putting GenAI to work in software development. Priorities center on software/product innovation (49%), enhanced focus on business objectives and outcomes (47%), recommendations, decisions and predictions (46%), automation of tasks from end-to-end (45%) and a DevSecOps approach that incorporates more security (45%). These drivers correlate to broader trends in DevOps, including the fact that broader and more mature deployments tend to focus on business objectives and outcomes in addition to technical ones, and that security and DevSecOps have emerged as priorities, not just for security teams but also those involved in software development and deployment.
Want insights on DevOps trends delivered to your inbox? Join the 451 Alliance.