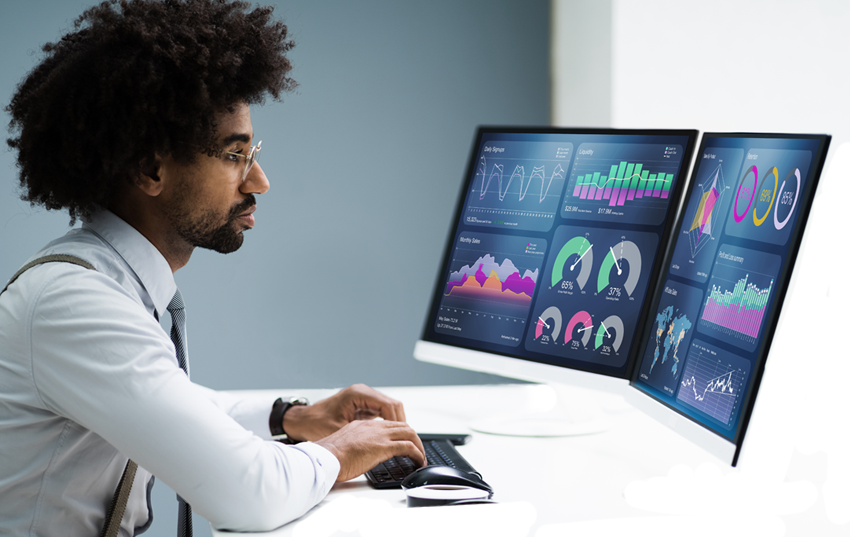
Ensuring data quality is mission-critical for organizations to achieve insightful analysis that aligns with their business strategies. A preventive approach calls for data observability, a methodology rooted in software engineering, to identify and assign corrective action for anomalies in near real time, writes Paige Bartley, Senior Research Analyst, data management, at 451 Research.
Garbage in, garbage out
Data-driven organizations are bound to deal with quality issues as data volume grows amid an increasingly complex and diverse enterprise data ecosystem. Nearly half (47.7%) of respondents in the 451 Alliance Data Management & Analytics survey affirmed that data quality was a major analytics challenge.
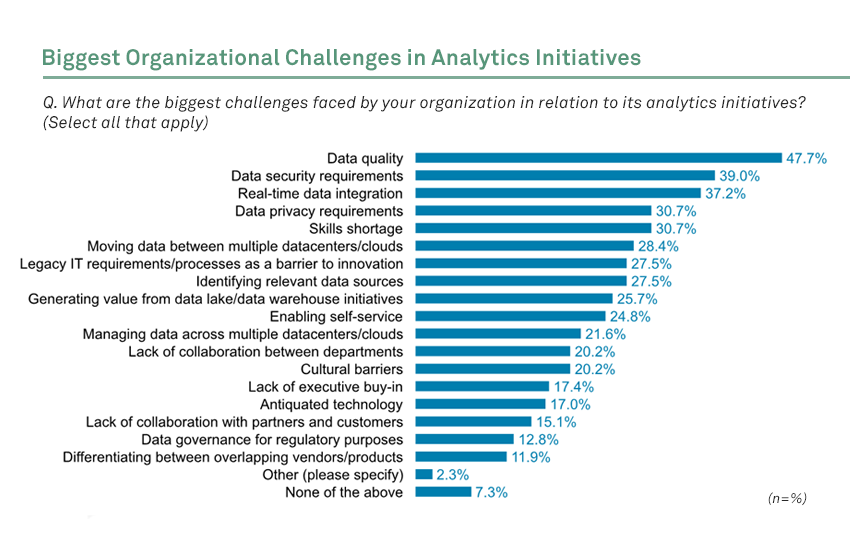
Poor data quality can lead to misguided solutions that potentially can be more damaging than insights derived from qualitative information or experience. The effect compounds over time, while the more established organizations are doubly burdened with the challenge of remediating both the historical and contemporary data.
Prevention over remediation
Early detection of potential data system anomalies saves time and effort. This is where data observability can identify and fix potential outages before they happen. The data quality monitoring workload should be moved upstream, to the data engineers who are already involved with the construction and maintenance of data pipelines, as well as the delivery of data supply.
This approach is borrowed from the software engineering methodology that seeks to continuously monitor the material output of data systems to infer their overall performance and health. Early intervention could minimize the risk of poor integrity data being sent downstream to data consumers, who may be less well trained to recognize and address the data quality issue.
Nevertheless, the observability approach does not obviate the need for traditional data remedial efforts. Organizations dealing with historical data may still have to perform cyclical assessment and remediation of data quality for as long as they need to leverage these data sets.
Quality solutions and key players
There remain two subsegments in the data quality market – those that focus on early detection of anomalies and outages, and the other camp that deals with remediation of existing quality issues.
At the same time, algorithmic data quality scoring is an increasingly common embedded feature in data management and data analytics platforms for contemporary data.
A one-size-fits-all method is not very likely. The data quality market offers complementary functionality and synergies across providers that focus on early detection and remediation effort.
Some key players in data observability are:
- Anomalo
- Bigeye (formerly Toro)
- Databand
- Datafold
- Lightup
- Monte Carlo
- Soda
The automated future
Development of automation technology may offer potential to the data observability subsegment, as sophistication of embedded machine learning functionality will enhance detection of potential issues and outages.
Want insights on data platforms and analytics trends delivered to your inbox? Join the 451 Alliance.