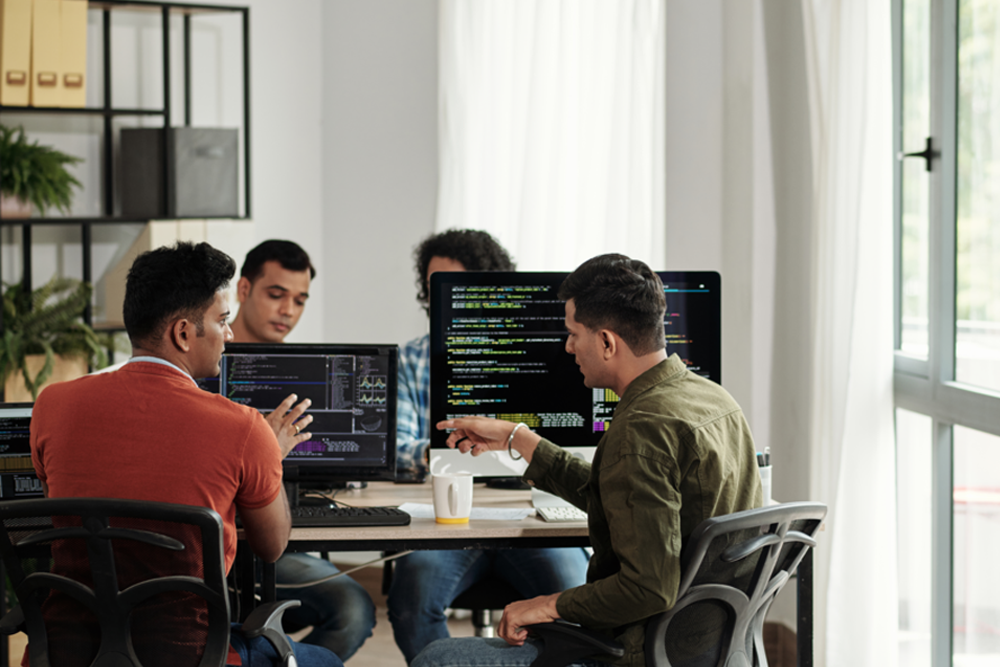
The rapid early growth of foundational model companies such as OpenAI is promising, prompting a frenzy of dealmaking in this field. What is less compelling is the real business value of foundation models, as customers are ultimately seeking ready-to-use solutions, writes Nick Patience, managing analyst at 451 Research, a part of S&P Global Market Intelligence.
Deals, deals, deals
Generative AI, the technology that mines data to write codes, college essays and create images impressed the public and the media when OpenAI released ChatGPT 3.5 in late 2022. Nine of the top pure-play foundation model companies—AI21 Labs, Aleph Alpha, Anthropic, Cohere AI, Hugging Face, Inflection AI, Mistral AI, OpenAI and Stability AI—raise $28.67 billion in disclosed rounds, according to S&P Global Market Intelligence. Aside from pure-play firms, the market includes hyperscale cloud vendors, tech vendors like IBM, social media firms such as Meta Platforms and specialist vendors with tools to generate various data types.
The huge outlay underlines the race to secure dominance in this emerging field, led by AWS, Microsoft and Google, who in turn provide cloud infrastructure and access to chips for these startups to train the models.
Value creation
While models are important, they are not the application itself. Additional elements are required to enable models to solve complex business problems. One approach is retrieval augmented generation (RAG), which supplements foundation models without the need for fine-tuning. RAG leverages vector databases to store, index, and process high-dimensional vectors representing unstructured data.
However, the search lookup process must perform well to avoid slowing down and compromising the user experience. RAG 2.0 advocates for better precision and recall, and chaining methods connect multiple prompts to generate complex content by breaking down tasks into smaller parts. Complexities arise when a retrieval engine works across different models and modalities, as they impose different pressures on infrastructure.
The real test
The field of MLOps (machine learning deployment and operations) is expanding into GenAIOps to address the increasing complexities and novel security risks posed by GenAI. Unlike traditional predictive models, GenAI has less control over inputs and outputs, and the use of RAG presents data-related challenges. Therefore, besides the focus on larger and more performant models, other factors must be considered to ensure that these expensive models deliver real business value.
Want insights on AI trends delivered to your inbox? Join the 451 Alliance.