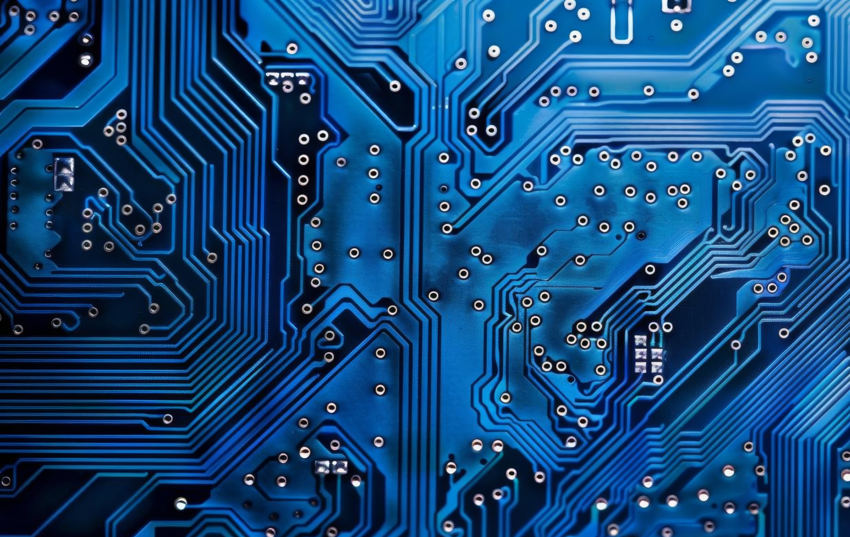
Source: nattapon98/Technology/Adobe Stock
The impact of recent news regarding the performance of generative AI models of the Chinese company DeepSeek was swift and pronounced. The sudden attention led to scrutiny of multiple aspects of DeepSeek — including its risks. The potential competitive threat is one of the major concerns, but security-, privacy- and technology-specific risks represent others.
The take
DeepSeek being a People’s Republic of China company attracted immediate attention, especially given that the data its self-hosted models collect is stored in the PRC under terms that reveal considerable ways in which that data can be shared — including with authorities — and under a privacy policy that provides limited detail on data retention. Not all DeepSeek models need be accessed online. The open distribution of DeepSeek’s models means that other service providers can potentially host them. The models’ low resource requirements mean individual or isolated instances may exist, and may not require network connectivity at all.
Researchers have assessed DeepSeek models for security issues. Early results indicate issues seen with other models, as well as security issues in the hosting infrastructure of DeepSeek’s own services, although some researchers see a higher incidence of issues specific to generative AI. Security professionals will have to evaluate these findings to be satisfied of their veracity. For now, the more serious threat DeepSeek poses seems to be to its competition, but the issues encountered so far are not likely to be ignored by the 58% of respondents that cited security and privacy concerns as the most frequently mentioned influence on large language model selection.
Policy
Among the first concerns identified were those explicit in DeepSeek’s privacy policy. In this policy, DeepSeek makes it clear that its self-hosted models collect a range of information, including personally identifiable data, technical information about the devices and networks used to access its services, usage information, cookies, and payments. The policy states that the uses for this information include compliance “with our legal obligations, or as necessary to perform tasks in the public interest, or to protect the vital interests of our users and other people.”
Raising concerns about such statements is a passage in that policy stating that, “We store the information we collect in secure servers located in the People’s Republic of China.” The policy further states that information may be shared with service providers, business partners, DeepSeek’s “corporate group” and others, including law enforcement agencies and public authorities, if DeepSeek has what it calls a “good faith belief that it is necessary to comply with applicable law, legal process or government requests” or to “detect, investigate, prevent or address … other illegal activity.” While these are not necessarily unusual terms in a privacy policy, it offers few specifics about items such as how long DeepSeek retains collected information, using statements such as, “We retain information for as long as necessary to provide our Services and for the other purposes set out in this Privacy Policy,” or “for as long as you have an account.”
DeepSeek also puts forward policies for terms of use, as well as terms of service for its distribution of its Open Platform. Among noteworthy passages from the terms of use: “DeepSeek will take necessary measures (not less than industry practices) to ensure the cyber security and stable operation of the Services.” In the case of security for generative models specifically, some of those practices may still be evolving.
Venue matters
It is important to note that DeepSeek’s access to the information provided to its models may depend on where and how those models run. Interacting with the DeepSeek-hosted service — which may include services provided to its apps for devices that access DeepSeek’s models over a network — may provide information to DeepSeek directly. However, DeepSeek also makes its models available for users or other service providers to run in their own environment, in a variant of open distribution known as “open weights.” In the case of personal or isolated deployment, the model may not require any network connectivity at all — and thus may not send, or may not be able to send, information to DeepSeek. DeepSeek’s memory and resource requirements for its distributable models are constrained enough that they can be run on sufficiently capable personal systems with no network connection — a factor that could contribute to making them popular for use cases at (or beyond) the network edge.
In this context, “weights,” as defined by the US Federal Trade Commission, means “the data that results from training and allows a model to generate content based on a prompt.” The concept of open weights differs from open source in that the source code of functionality is directly observable in the latter — including functionality that could pose security or other risks. In open-weights models, the model’s source code or other components may not be as visible for scrutiny, which serves to protect IP, but the weighting information can be applied and tuned for a specific use case.
The inability to analyze the model’s static components (its source code or other observable components that determine functionality) does not, however, mean that the behavior of locally running distributions of the model cannot be observed. In one recently publicized example, an analyst shared a screen in which a locally running copy of a DeepSeek model was asked what Tiananmen Square is known for. The model initially replied that it couldn’t answer the question, but with its DeepSeek-imposed guardrails defeated in a subsequent prompt, it was able to recount not only the history of Tiananmen Square, but also details about incidents of the 1989 pro-democracy protests. Service providers that run DeepSeek models in their environments may be able to adjust weights and modify guardrails more appropriately for their users, if consistent with applicable policies.
Observations of DeepSeek functionality have led some to believe that its models may have trained on those of others, using techniques such as unauthorized model distillation, in which “student” models are trained on the data from a “teacher” model that may have taken considerably more resources to train. It is a technique that could be leveraged to accelerate the race to competitive parity. OpenAI in particular has alleged that DeepSeek has trained on unauthorized distillation of its models. While it hasn’t yet provided detail on its evidence, reports indicate that Microsoft Corp. and OpenAI are investigating the potential exfiltration of large amounts of OpenAI data via user account access to an API in late 2024.
Other analysis of dynamic behavior can be observed in the runtime environment. Sandboxed environments exist to observe technology behavior for this specific purpose, and researchers performing such evaluations could possibly publish findings they deem noteworthy. In performing such analysis, researchers would bear in mind that there are distinctives in how AI models may run compared with other software. One variant is to run models within a platform such as Meta Platforms Inc.’s Ollama, which supports local execution of compatible third-party models without having to provide and configure complex resources to do so. Researchers examining behavior would need to distinguish the underlying model execution platform’s activity from that of the specific model in question.
Already, security researchers have assessed the publicly accessible surface of DeepSeek’s hosted models. Investigators with cloud-application-security company Wiz recently shared the findings of their analysis, in which they identified a publicly accessible ClickHouse database belonging to DeepSeek that, according to Wiz’s blog post on their findings, “allows full control over database operations, including the ability to access internal data. The exposure includes over a million lines of log streams containing chat history, secret keys, back-end details and other highly sensitive information.” The Wiz blog went on to note that the Wiz Research team “immediately and responsibly disclosed the issue to DeepSeek, which promptly secured the exposure.” While some have questioned the ethics of performing such a scan without the prior cooperation of the subject, this discovery points out an issue not unique or even specific to AI deployments. Securing the technology stack is a fundamental for any publicly exposed application. In the rush to get compelling and competitive features to market — particularly in an aggressive space with the perceived potential of AI — lapses in securing the overall environment could have consequences.
Other researchers have put DeepSeek to the test as well. Palo Alto Networks Inc.’s Unit 42 researchers subjected a DeepSeek model to generative AI jailbreaks they call Deceptive Delight, Bad Likert Judge and Crescendo. In a blog post about the effort, they note that they “tested against one of the most popular and largest open-source distilled models,” further noting that they “have no reason to believe the web-hosted versions would respond differently.” Not only did they achieve “significant bypass rates, with little to no specialized knowledge or expertise being necessary,” they were able to have the model produce “specific and comprehensive guidance” on attack techniques including data exfiltration, spear phishing and social engineering.
Comparisons
It is important to note that a wide range of models have already been subjected to security researcher evaluation, notably in efforts such as the DEF CON AI Village Generative AI Red Team initiative. Most, if not all, have exhibited various security issues when subjected to such evaluations, indicating that it is still early days for the field of security for AI, which is likely to develop further — and quickly — given AI’s rapid evolution.
That said, some evaluations of DeepSeek have been comparative, but the nature of those comparisons must be understood to be evaluated. In its report on its DeepSeek security assessment, security-for-AI company Enkrypt AI claimed that DeepSeek R1 was three times more biased than claude-3-opus, four times more vulnerable to generating insecure code than OpenAI o1, four times more toxic than gpt-4o and 11 times more likely to create harmful output than o1. Such reports must be studied for detail on these comparisons — indicating the degree to which security professionals will have to invest in such research to become literate in evaluating claims, not only from model providers, but also from researchers. For example, in the case of the Enkrypt analysis, the report says that 78% of attacks against the DeepSeek model were successful in generating insecure code. This report, however, does not go into detail on the specifics of test attacks, the testbed and evaluation scenarios. Security professionals looking to validate such findings may press investigators for this level of detail, in order to identify analysis most applicable to real-world deployments.
Conclusions
Given our survey findings, it seems likely that organizations may take a conservative view of embracing the models of a company that gathers large amounts of a wide variety of information — especially if that company retains that data in the PRC. The converse could also be felt by non-US companies doing business with US-based models — or with models in any venue where the customer has limited or no visibility or control. Such an entity doesn’t even need to be outside the customer’s geography to be a concern. As adversaries add to their arsenals of ways to exploit generative AI (Google researchers recently updated their enumeration of ways that generative AI could be attacked), those concerns could grow in the wake of incidents once they begin to appear.
Unless and until such incidents challenge its perceived value, the advantages of cost and performance imputed to DeepSeek so far have been an even greater disruption to the AI landscape — for now.
Want insights on Infosec trends delivered to your inbox? Join the 451 Alliance.