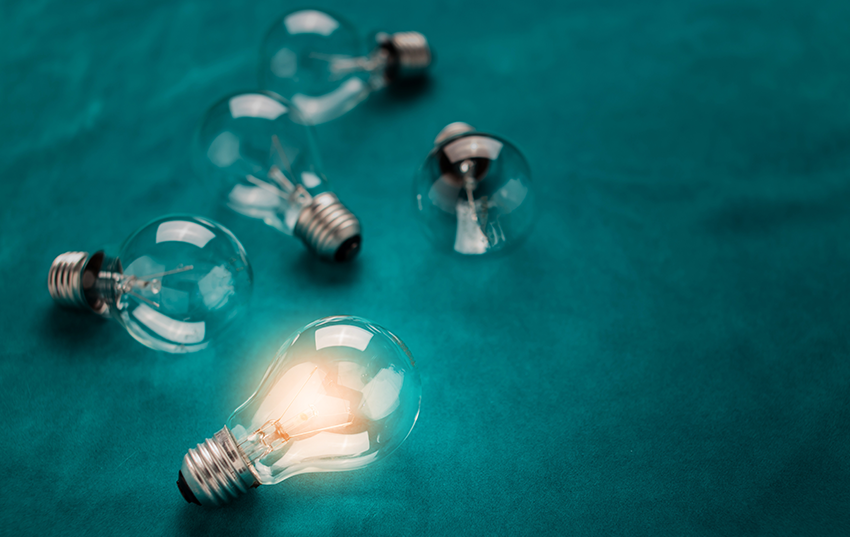
Source: whyframeshot/Business via AdobeStock.
Agentic Artificial Intelligence (AI) stands at the forefront of technological advancement, promising to revolutionize how organizations operate by shifting the focus from passive information processing to active problem-solving. In part 1 of this two-part blog post series, we explored the concept of agentic AI as the next frontier in generative AI, highlighting its potential to revolutionize productivity and revenue streams. In part 2 we’ll delve into the evolving ecosystem and future trends in AI consumption models.
Practical implementation of agentic AI
Agentic AI, with its potential to transform digital workflows, requires careful planning and execution. Here’s how organizations can navigate this complex landscape:
- Assess needs and goals: Begin by clearly defining the objectives and expected outcomes of deploying agentic AI. This involves understanding the specific problems AI agents are expected to solve and the value they are anticipated to bring. A well-defined goal helps in selecting the appropriate tools and frameworks that align with organizational needs.
- Choose the right architecture: Depending on the complexity and requirements of the tasks, organizations can choose between different agent frameworks. ReACT agents, known for their customizability, are ideal for sophisticated applications that require structured reasoning. Function calling agents, on the other hand, offer a more direct and efficient approach for scenarios where rapid decision-making is crucial. In some cases, a hybrid approach may be beneficial, combining the strengths of both types.
- Invest in modular systems: Moving away from monolithic AI models to modular systems is essential. Modular AI systems break down complex tasks into smaller, manageable components, enhancing scalability and efficiency. This approach allows for more sophisticated interactions and better handling of complex workflows, making it a critical strategy for implementing agentic AI.
- Develop robust training data: The accuracy and reliability of AI agents heavily depend on the quality of training data. Fine-tuning AI models with relevant and specific data ensures that the outputs are accurate and aligned with organizational needs. Techniques like retrieval augmented generation (RAG) can further enhance the model’s ability to provide relevant and factual information.
- Pilot and iterate: Before full-scale deployment, organizations should start with pilot projects. This allows for testing and refining AI agents in a controlled environment, gathering insights and making necessary adjustments. Iterative testing helps in identifying potential issues and optimizing performance before broader implementation.
Governance challenges
While the potential of agentic AI is immense, it brings significant governance challenges that organizations must address to ensure ethical and safe use. As AI agents become more autonomous, robust governance structures become essential to manage risks and ensure compliance. Key governance considerations include:
- Accountability and bias: As AI agents make decisions and take actions, establishing accountability is crucial. Organizations must implement clear guidelines for agent behavior to prevent bias and ensure decisions are fair and transparent.
- Security and compliance: Ensuring strong security measures and compliance with regulatory standards is vital. This protects against misuse, data breaches, and vulnerabilities that could compromise the integrity of AI systems.
- Regular audits and monitoring: Continuous monitoring and regular audits of AI systems are necessary to maintain performance and ensure adherence to established guidelines. This proactive approach helps in identifying and mitigating potential risks.
- AgentOps practices: Extending MLOps principles to AgentOps focuses on the lifecycle management of AI agents. This includes training, deployment, and ongoing management, ensuring that AI agents remain effective and aligned with organizational goals.
By addressing these governance challenges, organizations can harness the transformative potential of agentic AI while minimizing risks and ensuring ethical use. Staying informed and proactive in both implementation and governance will be key to leveraging the full capabilities of these advanced technologies.
Want insights on AI trends delivered to your inbox? Join the 451 Alliance.
Notice and Disclaimer: Some of the content in this 451 Alliance blog leverages artificial intelligence in accordance with our Terms of Service.