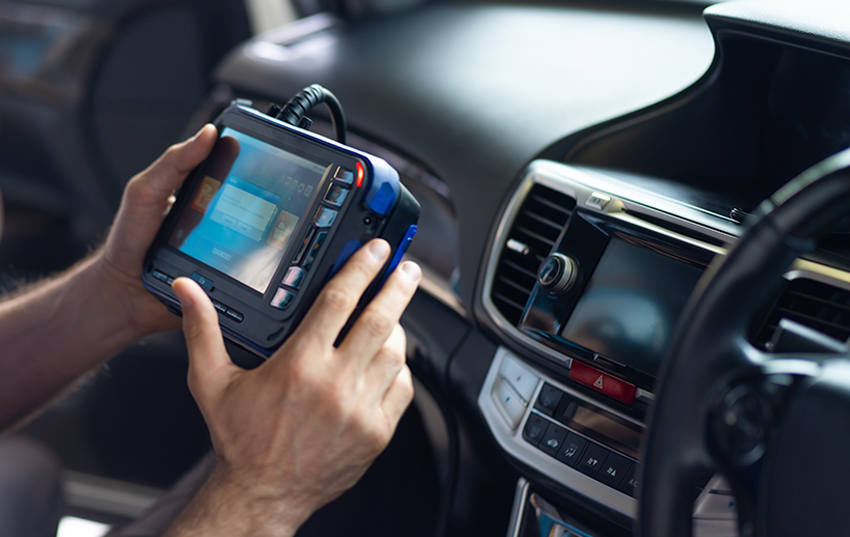
Source: NVB Stocker/People via Adobe Stock.
The automotive sector is experiencing a significant change as it transitions from being focused on hardware to embracing software-defined vehicles. In part 1 of this two-part blog post series, we provided some context around open-source software’s vitality in the automotive sector and provide examples of use cases. In part 2, we’ll be discuss artificial intelligence (AI)’s role in automotive open-source software and sharing some guidelines to promote digital infrastructure success.
AI’s advancements in automotive open-source software
The combination of AI and open-source software is propelling advancements across multiple domains, including autonomous driving and manufacturing. This integration not only improves the development process but also enhances the overall user experience. A notable example is CARLA (Car Learning to Act), an open-source simulator developed by the Computer Vision Center at the Universitat Autònoma de Barcelona, in partnership with Intel Labs and the Toyota Research Institute. CARLA is specifically designed for autonomous driving research, providing realistic urban environments, vehicles, and sensor suites to support the development, training, and validation of autonomous driving systems.
The SOAFEE Reference Implementation leverages AI to enhance advanced driver-assistance systems (ADAS) and autonomous driving capabilities. Similarly, Eclipse ADORe utilizes AI for decision-making, planning, control, and simulation in automated vehicles.
Within vehicles, AI significantly enriches the driving experience by powering infotainment systems, driver-assistance features, and predictive maintenance. The Automotive Grade Linux Unified Code Base facilitates AI applications that improve user experiences and vehicle functionality. Additionally, the Connected Vehicle Systems Alliance is dedicated to developing open-source solutions for in-vehicle infotainment systems, which may incorporate AI-driven features.
AI is also revolutionizing automotive manufacturing and design processes. AI-powered tools and machine-learning algorithms contribute to improved production efficiency, optimized supply chains, and enhanced vehicle design. For example, AI systems can expedite data classification in risk assessments and vehicle damage evaluations while automating repetitive tasks in manufacturing. Companies such as NVIDIA Corp. and Rethink Robotics are harnessing AI to refine design workflows and develop collaborative robots tailored for the automotive sector.
Guidelines for automotive digital infrastructure success
Automotive digital infrastructure providers can benefit from open-source solutions, which offer flexibility and customization. This approach streamlines development processes and allows for system tailoring without the limitations of proprietary software. It enhances scalability, reduces costs by lowering licensing fees, fosters collaboration, and facilitates the integration of new technologies.
Recommendations:
- Strengthen security and compliance: Providers should implement strong security measures and adhere to industry standards. Utilizing community-driven security updates and best practices can help mitigate risks.
- Engage with the community: Active participation in open-source communities can spur innovation and provide access to diverse expertise. Collaborating with these communities can lead to the creation of more robust solutions.
- Invest in training: Offering training programs for developers and IT staff on open-source tools can improve their proficiency and effectiveness.
- Form strategic partnerships: Collaborating with other organizations in the open-source ecosystem can enhance resource sharing and knowledge exchange, accelerating technology development and system integration.
- Adopt best practices: Implementing best practices from successful open-source projects can enhance project management and efficiency. This includes using version control, continuous integration/deployment, and automated testing frameworks.
- Ensure interoperability: Open-source solutions should be compatible with existing systems and other open-source projects. This can be achieved by following open standards and protocols.
- Maintain continuous homologation: It’s essential to ensure that open-source software in vehicles complies with safety and cybersecurity standards. This involves tracking changes, utilizing community testing for robustness, certifying contributions, and securing over-the-air updates.
Want insights on IoT trends delivered to your inbox? Join the 451 Alliance.